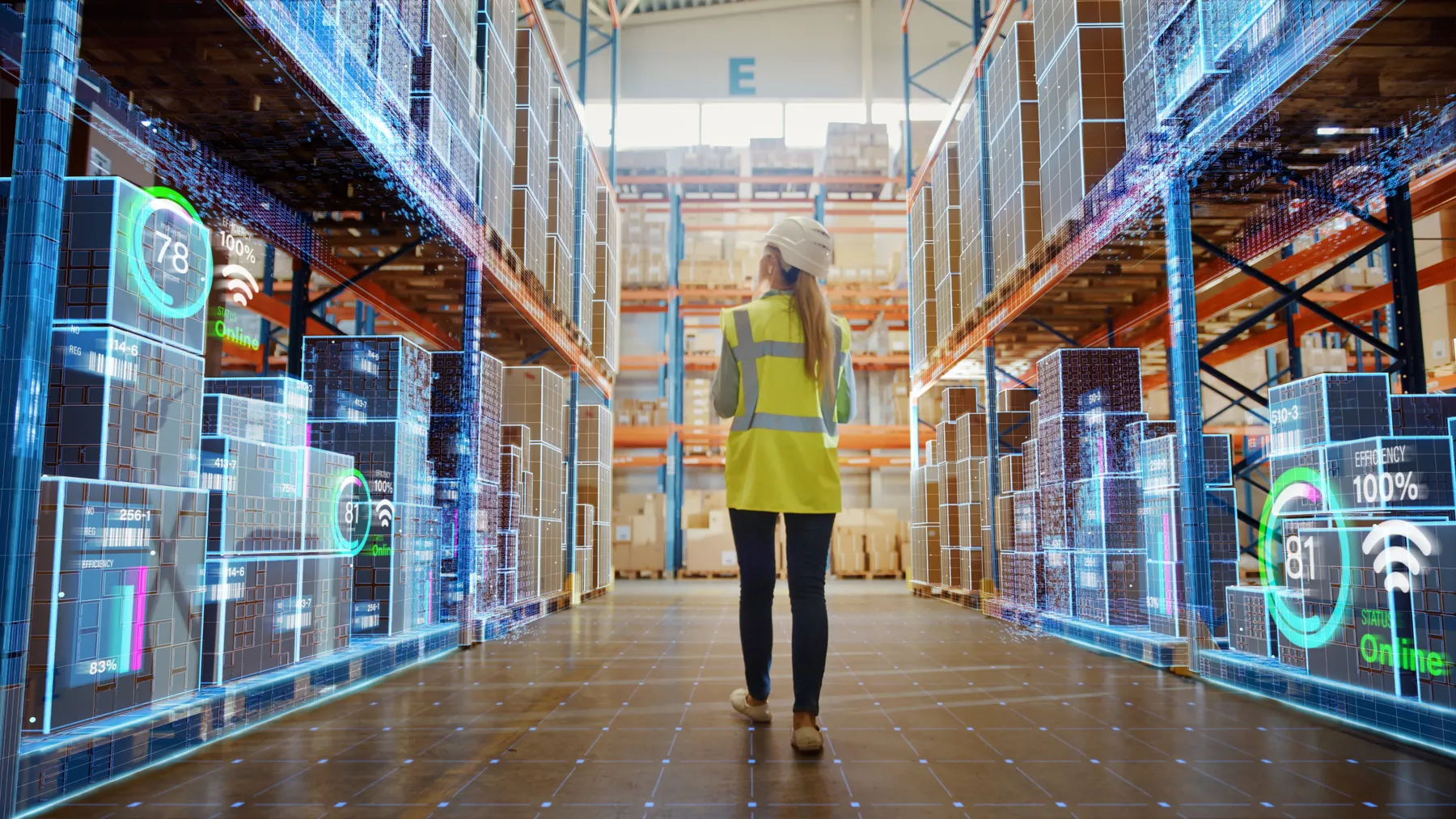
AI opens new frontier in supply chain planning
The year 2023 broke the crystal balls.
Some of the largest, most sophisticated companies in the world overbought or overproduced inventory by many millions of dollars. The reason, at its simplest, is that consumers did not behave as predicted.
Planners don’t have the luxury of blaming consumers for not acting according to their forecasts — especially when many of those customers were reacting to historic inflation in food, fuel and housing.
“Demand planning went out the door for three years,” said Rick Jordon, senior managing director with FTI Consulting. “Now they’re picking it back up, because they have all this excess inventory everywhere. And they’ve forgotten how to do it, or they didn’t do it well before.”
After three years of pandemic disruption and economic volatility, sharpening demand forecasts and inventory planning across industries seems both as imperative and challenging as it has ever been.
To get better results, companies are turning to new data sets and new technological tools like artificial intelligence to analyze them.
From spreadsheets to AI
An executive survey by McKinsey from early 2022 found that spreadsheets are still the primary planning tool for supply chain leaders, with 73% of respondents relying on them.
But, 43% said they planned to use artificial intelligence and machine learning for some planning activities. Another 17% said they planned to use AI and machine learning for most activities at some point.
A 2023 from BlueYonder, a supply chain software company, found that demand forecasting and inventory optimization are among the top uses of AI and machine learning technologies by supply chain executives
“We're moving well beyond Excel, and just moving to a more advanced tool set,” Ron Scalzo, senior managing director with FTI Consulting.
In Jordon’s view, AI technologies will at some point help remove human bias from forecasting and lead to more systematic predictions, as well as run multiple predictive scenarios at the same time.
Jordon noted that AI is needed to process through many data sets and help make sense of a company’s own internal data. “Most organizations have a lot of data,” Jordon said. “Are they using that to the best of their ability, utilizing the best technology and ignoring that noise? That’s really where the next three or four years of development will happen.”
Finding the right data to analyze the right way
At the most basic level, AI takes in data and spits out predictions. To get the best predictions, the data fed into them might matter even more than the AI tools themselves.
“There are systems pulling in data which is lying inside the company already, from across their enterprise systems, POS systems, the inventory levels from their stores and all that,” said Meher Dinesh Naroju, director of AI services at Centific. “There are very few companies that actually go outside of this box and get data from sources that are completely unstructured.”
Such data sources include things like social media, which can provide clues about burgeoning market trends. Customers are “actively engaging and leaving opinions and clues about various things they intend to do in the market or a specific category,” Naroju said.
Even images from a retailer’s store cameras might house data relevant to customer behavior in the aggregate, including reactions to certain products, brands and categories. “It’s a long shot, but it can help,” Naroju said.
Other sources, such as weather data, can provide early hints about supply chain timelines and inventory arrivals, he also noted.
Still, all of these data sets have to be put into the context of local and regional cultures and other marks of differentiation.
“It is of the utmost importance to pick and choose what data you need and very clearly segment the data you want to analyze,” Naroju said.
Once a company has the best, most refined and useful data it can get, that’s where AI tools become critical. The technology can derive insights from large pools of unstructured data that “no human being can do in so short a time,” Narajou said.
But even with the most creatively sourced data and advanced tools available, a prediction is still just a prediction.
“If you crack the code on a perfect forecast with the consumer today, you're quickly going to be the richest person in the world,” said Jake Self, vice president of operations for third-party warehousing and logistics company Smart Warehousing.
‘So much noise’
Businesses for generations have relied on the past to help them anticipate the future. That may seem outdated after the pandemic and then inflation scrambled the usual patterns, with volatile cycles piggy backing on each other since 2020.
But for many, historical trends are still the most useful forecasting data. “It’s not especially sexy, but we’ve actually philosophically been using the perspective that history is the best predictor of the future,” Destination XL Group CEO Harvey Kanter told Supply Chain Dive in an April interview.
“I think there's been so much noise in the last three-plus years that the roller coaster we’ve been on is almost impossible to predict,” he added. “I don’t know that the greatest level of AI and ML can predict what the consumer is going to do.”
Kanter went on to note that the apparel retailer still looks at pre-pandemic revenue and historical revenue contributions by day, week and month over the past 10 years, which he said has remained remarkably consistent.
Other businesses simply don’t have the budget to pay for or justify advanced technological tools. Smart Warehousing’s clients run the gamut from small brands operating out of their garages to Fortune 500 companies. For some of the smaller companies looking for replenishment and warehousing help, their forecasting process amounts to “hopes and dreams,” Self said.
For those companies, historical data is by leaps and bounds better than no data. “We've spent a lot of time on our replenishment program, really putting an algorithm in place that learns and understands based on historicals where we're going to need to be,” Self said.
Smart Warehousing can do that having invested in forecasting and replenishment technologies for more than two decades, and with an entire team of specialists dedicated to that one skill set. An algorithm for cold replenishment operations out of Missouri “looked like something out of Good Will Hunting,” Self, referring to the film that features actor Matt Damon playing an unknown mathematics prodigy.
But even with those resources, prediction is still tough in the current environment. “If you're not planning for an element of the unexpected, then you're probably not planning right,” Self said.